Category: Uncategorized
Organization of Research Data and Software team, TU Delft Library
The Research Data and Software (RDS) team at the TU Delft Library has been undergoing an extensive grow during the last half an year. With the last new team member starting in mid-January 2024, we are very proud to present the complete team organization. For more information on the Research Services department at TU Delft Library, please visit this blog.

Research Data Management & Digital Skills Training
The training team is led by the coordinator and consists of 4 trainers, 1 educational advisor and 1 training assistant.
- They implement and update the ‘Vision for Research Data & Software management training at TU Delft’.
- They coordinate, organise and run training for PhD candidates and researchers (and MSc students in some cases)
- They collaborate with stakeholders from library, TU Delft and external on new training development and integration.
Data Stewardship
RDS provides the coordination of the TU Delft Data Stewardship. The Data Stewardship coordinator and the library Data Steward are in the Data Stewards team together with all faculty Data Stewards.
- The coordination is twofold: coordination within the Data Stewards team and coordination with all relevant university service teams.
- The Library Data Steward facilitates on all innovation projects within the Data Stewards team, and also provides the functional management of related applications (DMPonline, ELNs).
Digital Competence Centre
The RDS team is part of the TU Delft Digital Competence Centre (DCC) in collaboration with ICT innovation. Four data managers from RDS work are members of the DCC team and provide hands-on support on open and FAIR data management to researchers. The daily operation of the DCC team falls under the coordination of DCC coordinator from ICT innovation.
Innovation
The RDS team runs innovation projects which aims to pilot new services/applications, explore solutions to emerging issues or address future directions in the domain of research data and software management. Three current projects are supported by the TU Delft Open Science program:
- iRODS pilot (collaboration with ICT innovation)
- Guidelines for research data and software management in various research fields
- Research data and software peer review (collaboration with TU Delft OPEN Publishing)
Student assistants
Last but not the least, we have 2 brilliant student assistants working with the team members on updating content information on the Research Data Management (library website) and organizing various training events.
2023 Wrapped!
Author: Esther Plomp
After writing overviews for 2021 and 2022, I decided to wrap 2023 up in a different way! If you’re not a Spotify user the overview might look a bit… interesting:
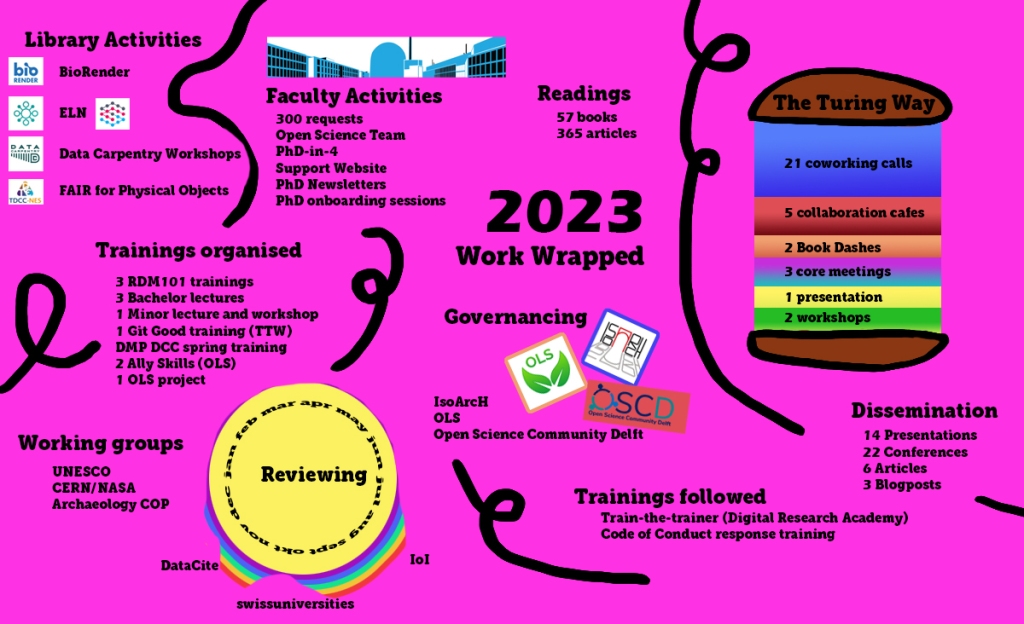
Some more details:
Faculty Activities
- 300 requests
- Open Science Team
- PhD-in-4
- Open Science Support website now has 105 posts! (Not all are finalised and can be indicated with WIP – work in progress)
- 12 PhD newsletters (bringing us to 46 in total!)
- 2 PhD onboarding sessions
Library Activities
- BioRender
- Electronic Lab Notebook working group and key user for eLABjournal and RSpace
- Data Carpentry Workshops
- Workshop on FAIR for Physical Objects – Summary and Conclusions
Trainings
- 3 RDM101 trainings
- 3 bachelor trainings (ME and EUR)
- Lecture and workshop for nano minor
- 1x Git Good training (TTW)
- DMP DCC spring training
- 2 Ally Skills for OLS
- 1 OLS project
Trainings followed
- DRA – Train-the-trainer event (see Reproducible and FAIR Teaching Materials)
- Code of Conduct response training
The Turing Way
- 20 coworking calls
- 5 collaboration cafés
- 2 Book dashes (see blogpost)
- 3 Core meeting
- 1 presentation (csv,conv,7)
- 2 workshops for two conferences (Open Science Conference / AI UK)
6 articles/reports published:
- Ten simple rules for starting FAIR discussions in your community
- A Manifesto for Rewarding and Recognizing Team Infrastructure Roles
- A Guide for Social Science Journal Editors on Easing into Open Science
- Faculty of Applied Science and Open Science – A team: Overview 2021-2023
- Valuing a broad range of research contributions through Team Infrastructure Roles: Why CRediT is not enough
14 Presentations/panels
- 10 simple rules for starting FAIR discussions in your community, Jülich Open Science Speaker Series
- Five selfish reasons to join an open science initiative, Open Reproducible Data Science and Statistics network in MV
- Open Data, Open Seeds / OLS-7
- Working towards an open, collaborative and reproducible data culture in
- Rewarding and recognising Team Infrastructure Roles, csv,conf,v7
- VU International Women’s Day 2023
Working Group meetings
- UNESCO groups (Capacity Building, Open Science Policy, Monitoring)
- Archaeology CoP meetings
- NASA/CERN groups on (Sustainable & Open Infrastructure, Evidence-based OS policies)
Review activities
- IOI: Open Infrastructure Fund
- Swissuniversities: Open Access and Open Research Data Calls
- Datacite: Global Access Fund
Board/governance activities
Conferences and events: 22
- Open Science Retreat
- Rewards and Recognition Festival (session on recognising supporting roles!)
- Csv,conf,7
- OSFNL (with sessions on an Open Science Event Playbook and Peer Reviewing datasets)
- Dutch Reproducibility Network Launch
Articles read: 365 total with some of my highlights listed here:
- 17 / On the long-term archiving of research data
- 25 / Framing Power: Tracing Key Discourses in Open Science Policies
- 33 / Open Science at the Montreal Neurological Institute and Hospital: the buy-in process
- 37 / The critical need to foster computational reproducibility
- 119 / Why do we do science? Navigating the paths of individual excellence and #TeamScience
- 122 / Redesign open science for Asia, Africa and Latin America
- 129 / The Invisible Workload of #OpenResearch
- 140 / ASAP Blueprint for Collaborative #OpenScience
- 143 / Observing many researchers using the same data and hypothesis reveals a hidden universe of uncertainty
- 150 / Against management: Auto-critique
- 151 / Data management in #anthropology: the next phase in #ethics governance?
- 175 / Understanding the Impact of Equitable collaborations between Science Institutions and #Community Based Organizations: Improving Science through Community-Led Research
- 191 / Evaluating Academic Scientists Collaborating in Team-Based Research: A Proposed Framework
- 200 / A reality check on research reproducibility in Open Science student’s projects
- 201 / Agency, Scale, and the Ethnography of #Transparency
- 203 / Making #Qualitative Data Reusable A Short Guidebook For Researchers And #DataStewards Working With Qualitative Data
- 212 / Article processing charges for open access journal publishing: A review
- 220 / The manifold costs of being a non-native English speaker in science
- 285 / Actually Accessible Data: An Update and a Call to Action
- 286 / Open (for business): big tech, concentrated power, and the political economy of Open AI
- 291 / Understanding the Data Management Plan as a Boundary Object Through a Multi-Stakeholder Perspective
- 300 / A systematic scoping review of the ethics of Contributor Role Ontologies and Taxonomies
- 306 The #SCOPE framework – implementing the ideals of responsible #ResearchAssessment
- 327 / A snapshot of the academic #ResearchCulture in 2023 and how it might be improved
- 330 / Navigating Risk in Vendor Data Privacy Practices: An Analysis of Elsevier’s ScienceDirect
- 343 / The Oligopoly’s Shift to #OpenAccess. How the Big Five Academic Publishers
Profit from Article Processing Charges - 348 / The evaluation of scholarship in academic promotion and tenure processes: Past, present, and future
- 350 / University professional staff roles, identities, and spaces of interaction: systematic review of literature published in 2000–2020
- 352 / The #Qualitative Transparency Deliberations: Insights and Implications
- 353 / The neglect of equity and inclusion in #OpenScience policies of Europe and the Americas
Books read: 57 total
- Wheel of Times series!
- From here to eternity by Caitlin Doughty
- Counting by Deborah Stone
- How to work with (almost) anyone by Michael Bungay Stanier
- Conflict is not abuse by Sarah Schulman
- Avoiding anxiety in autistic adults by Luke Beardon
- The choice by Edith Eger
Things I did not manage to do in 2023
- Did not engage a lot with the Faculty Diversity board (2024?)
- Did not check in with research infrastructure roles at my own faculty (2024?)
- Did not develop a course for data/code sharing for the PhDs (2024?)
- Did not start a new sport (and will probably give up on that idea… :))
Looking forward to continuing my activities after a long holiday – see you in February 2024! Happy Holidays!
Making Priorities for Digital Sovereignty
Discussions at the Leiden University & Elsevier Symposium on Digital Sovereignty, held on 29 November, illustrated how complex yet necessary this relatively new concept of digital sovereignty is. Even the representative from Microsoft, was convinced of the importance of the term. For him, it is a way for gaining and securing customers’ trust, privacy and security for the digital services they provide (and, of course, making a profit)
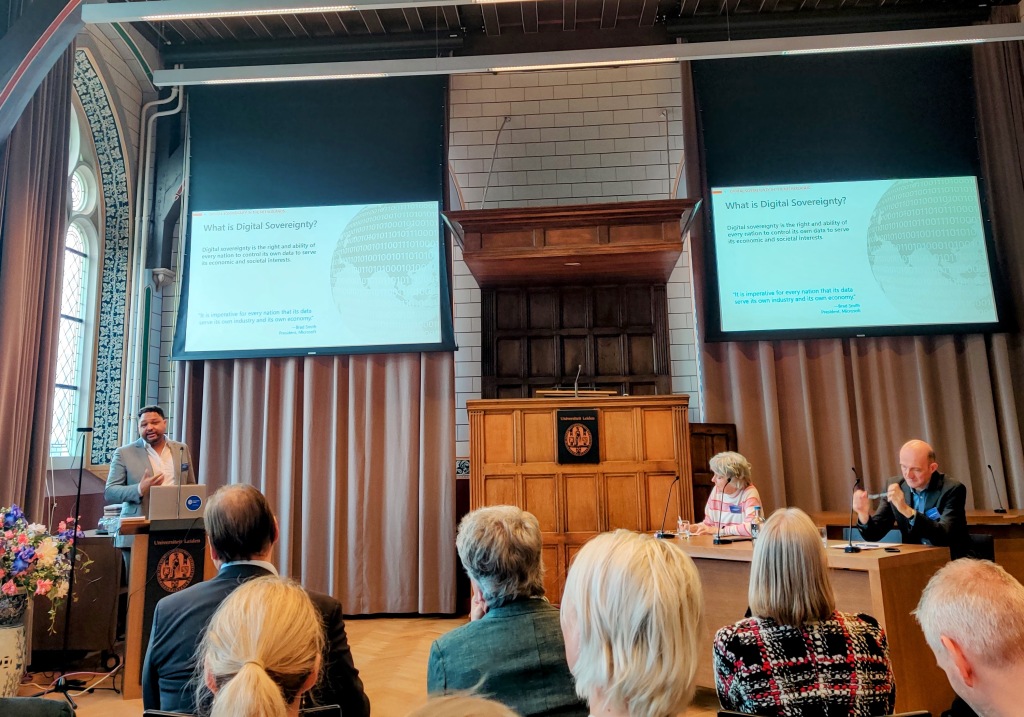
Azar Koulibaly from Microsoft giving his presentation on ‘Public Values in the Digital Age: Challenges and Opportunities for the Netherlands‘
In the Dutch university sector, we already have some projects that are inspired by the broader discussion over digital sovereignty. There is the Public Spaces movement, largely initiated by the broadcasters, but with some interest from higher education. Surf is making various eye-catching projects, such as its own Large Language Model, and has started an Open Research Information Agenda. And 4TU.ResearchData’s Djehuty data repository software openly invites contributions from the research community. There are others.
But these are still relatively isolated steps.
Within the university / research library sector we are still missing the crucial conversation about how we can work together, and in what areas we want to prioritise.
As background context, the diagram by Bianca Kramer and Jeroen Bosman from 2019 remains a relevant depiction of the landscape of research tools. (their related slides anticipate nearly all of this blog post). It does not depict all the digital services that university researchers make use of it – far from it – but it nevertheless highlights the complexity.
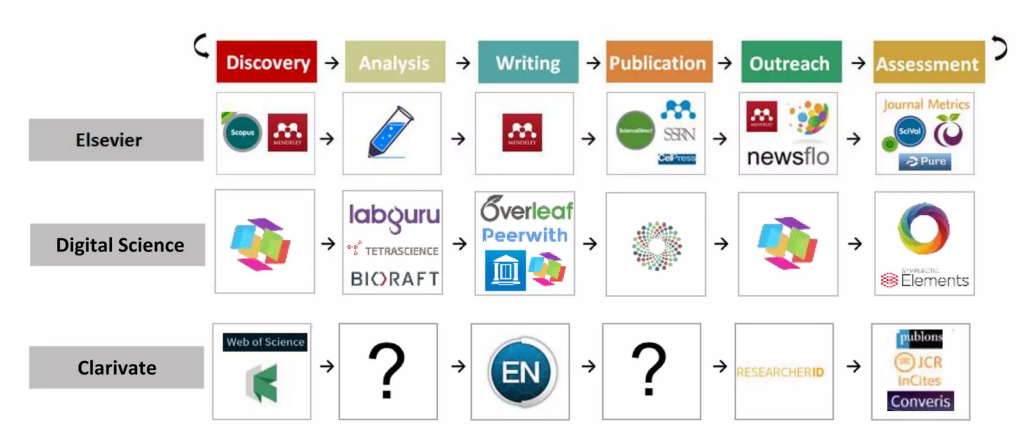
Most importantly, the diagram demonstrates the various arenas within the researcher lifecycle where the university library sector have ceded (consciously or not) digital sovereignty to commercial actors.
Currently, most universities engage, in a contractual sense, with the providers of these servers in an individual context. University A does a deal for Dimensions, and University B does a deal for Mendeley. This is opposed to deals done for access to ‘traditional’ publishing, where the Dutch universities have a history of organising collective agreements with the larger publishers
It seems to me that there is plenty of opportunity to work together to deal with this challenge more effectively: in the metaphor of speaker Ron Augustus from Surf, making honey by acting as bees in a colony.
Can we come together rather than work on this independently?
Some of the possible actions for a collective group might be:
- Defining the key areas on the researcher lifecycle (or indeed for any digital service) where we want to secure (or regain) our digital sovereignty
- Defining how we want to enact that digital sovereignty
- By continuing to have contracts with commercial players, but to do so at a collective level and informed by values (such as the Seven Guiding Principles for Research Information)
- By working together to create our own services to replace the commercial services
- By make sustained, strategic contributions to existing open source services at a global level (a simple example of which is the contributions made to the governance structure of the service Open Knowledge Maps). These contributions could be technical, financial or intellectual
- By making sustained, strategic contributions to open source networks such as SCOSS or Invest in Open
- And finally, defining what we mean by we. Universities? University Libraries? Medical Centres? Research Units? Or maybe even public sector organisations (broadcasters, museums etc)
As the Leiden symposium demonstrated, digital sovereignty is a problem you can drown in – overwhelmed by its size and complexity – and ending up going nowhere.
To avoid this, and to ensure our digital sovereignty, we need to make collective priorities.
Take-aways from Open Science Festival
Naomi Wahls, 1 September 2023
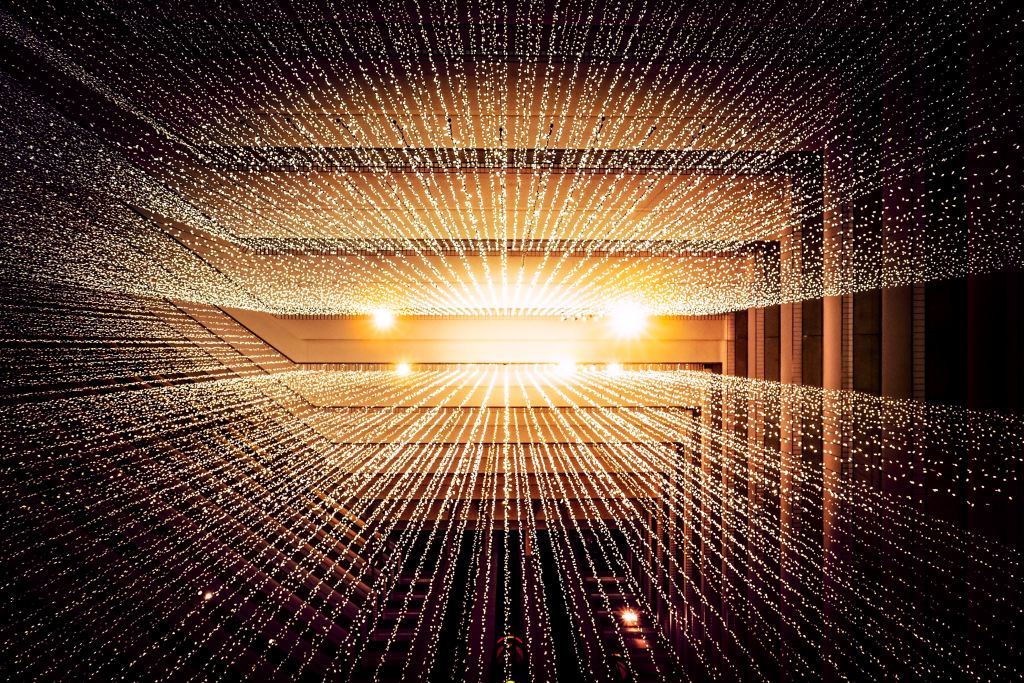
Photo by Joshua Sortino on Unsplash
The Open Science Festival was hosted by the Erasmus University in Rotterdam on 31 August 2023. Over 400 people registered for the event, ranging from experts in Open Science to those interested in learning about Open Science. It includes researchers, research support staff, and suppliers. The full programme includes presentations, plenaries, and networking time.
Reflection on Opening Session
The opening session was a nice confirmation of steps that TU Delft is taking in the right direction towards open by establishing Data Stewards and by creating grassroots funding opportunities for Open Science. The opening session was lead by Esther van Rijswijk and Antonio Schettino and included Annelien Bredenoord, Rector Magnificus of Erasmus University Rotterdam.
Open Science though tends to have more grassroot pilots in various stages with hopes of becoming embedded into an ecosystem. Before moving to the Netherlands, I supported virtual exchanges which often are grassroot pilots which hope to become embedded into an ecosystem between multiple universities. Due to my prior work, open education sparked my interest, particularly Open Educational Practice (OEP). Thus for me, Open Science feels like a more established infrastructure to empower research advancements. Ironically, Erasmus is globally known as a symbol of student mobility and thus is often the name leading virtual exchanges such as the Erasmus Virtual Exchanges.
Open Science events are an excellent space to see how others are collaborating and what advancements there are in the field. It’s also where you can foresee hurdles along the path of advancements.
The Open ebook from 2017 by Rajiv Jhangiani and Robert Biswas-Diener implied that OEP and Open Education overshadow Open Educational Resources (OER) which often take form in MOOCs in Open Education. For Open Science, the format of OER is often software such as a tool which some called Open Education Tools (OET) for a time, but that has seemed to disappear or reverted back to OER.
Session reflections
The first session I attended was Beyond Access: Making Research Discoverable, Accessible and Inclusive for All. This session speakers were Peter Kraker (Open Knowledge Maps), Astrid van Wesenbeeck & Martijn Kleppe (KB, National Library of the Netherlands), Maurice Vanderfeesten (VU University Library). To showcase the issue of paywalls, the first speaker was a primary school teacher who showcased the need for open access. In short, teachers do not have access that they might have had as a student through a university library. The example given was if the teacher reads a book with references that do not have open access, then they need to purchase subscriptions. A fundamental problem in the education system is that elementary and secondary schools do not have access to research that could provide professional development.
Following that, the struggle that the national library system has in improving services beyond creating awareness became more evident.
Next in that session was a demo of Open Knowledge Maps and then OpenAire. Both offer much potential to campus. Open Knowledge Maps seems to be a nice way to see what themes are in a repository or collection that we maybe didn’t notice yet. It could also be a nice way to allow end-users to make their own selection of items they want to display/save from our repository.
2.3 Connecting FAIR data with OPEN ACCESS Publications Project
This session was presented by Madalina Fron and Zahra Khoshnevis (TU Delft). The project was supporty by Yan Wang, Just de Leeuwe, and Frederique Belliard (TU Delft). This TU Delft initiative was a great starter project from Bachelor level students. They presented that 82% of peer-reviewed articles and 71% of conference papers are Open Access (OA), but that we lack numbers on open data. The project aims to review the connection between OA and Findable Accessible Interoperable and Reusable (FAIR).
The questions and answers posed in the session were difficult to follow by the audience, but were still relevant to the field. This session made me wonder how we train end-users for uploading and how we advise visitors of licensing and if there is room for improvement to embed training and advise in our repository further. The speakers brought up metadata and seemed to believe in sound metadata, but I also wonder based on the perception in the room what role automating metadata and checking and auto improving metadata is already expected from end-users. Perhaps current expectations are that showcased technologies at such events like these are already embedded into current tools.
3.4 Recognition & Rewards: Open & Responsible Research Assessment
This session was presented by Lizette Guzman-Ramirez, Nami Sunami and Jeffrey Sweeney (EUR). This final presentation offered an intriguing solution for recognition. They explained their recognition and rewards badging system and asked the audience for input on improving the badges. However, one audience member sparked a debate by asking if badges replace the current problematic system with another that simply repeats a checklist but offers a different list with different people giving the checks. The debate seemed to question the reasoning behind rewards and recognition. It also seemed to question how such a system relates to empowering researchers to advance their careers in a different way or provide transparency about their career opportunities. If we want to help researchers advance their career in a personalized way, could virtual career advice be applied to a researcher profile to improve the career opportunities based on their outputs, education, and other skills noted in a researcher profile? I wonder if something could be explored in that space in addition to the rewards and recognition programmes or as part of them.
Reflections on Open Education and Open Science events and Future Infrastructure Predictions
While I typically attend Open Education events, I’m new to attending Open Science events. Here are my reflections on Open Education events and future predictions.
Open Education events tend to be more about Massive Open Online Courses (MOOCs), although it is much debated if they are really massive since the original numbers of participants have decreased since the early years of MOOCs. In 2016, the trend in MOOCs predicted they would replace lifelong learning and provide flexible professional development (Castaño-Muñoz, Kreijns, Kalz, 2016). This prediction seems to be quite accurate. Willem van Valkenburg predicted in 2018 that “as more universities and higher education systems begin offering their degree programs online, we could be moving towards a single, global system of higher education” (The 9th TCU International e-Learning Conference in Thailand in 2018 (Pickard, 2018). I believe this prediction will also take place; it is only a question of when.
With these reflections in mind, I attended the Open Science Festival hoping to hear future predictions in the field and also hear how we’ve learned from the recent past, from predictions that missed the mark. I missed the closing due to last minute scheduling and thus missed the likely future predictions. The trends seem to be that the younger generation already assumed Open Science is a standard and they do not seem to be aware how much effort it took to get here. Across the field, there seems to be common support issues for Open-Source pilots seeking to become established services. Infrastructure for OER within Open Science is much needed. I look forward to future Open Science events and seeing the future advancements!
Archive Now! Your Data. Your Software. Your Knowledge.
Presentation given by Alastair Dunning at TU Delft Research Support Day, 26th September 2023.
Teaching Reproducible Research and Open Science Conference at Sheffield University
Last month (June 20-22), the trainer for Research Data Management and Digital skills from the Research Data and Software Team from TU Delft Library (RDS), Carlos Utrilla Guerrero was invited as guest speaker at Sheffield University in a three day ‘Teaching Reproducible Research and Open Science Conference’, to share our experience implementing the vision for Research Data and Software management training at TUDelft and teaching the RDM101 course, to help researchers develop the necessary skills to work as efficiently, reproducibly, and openly as possible.

The three-day event (20 June) kicked off with a symposium ‘Perspectives on teaching reproducibility’ organised by Sheffield Methods Institute (SMI) in collaboration with the University Library and Open Research Working Group (ORWG), and led by Aneta Piekut (SMI) and Jenni Adams (Library). The activities focused on incorporating reproducible methods in teaching reproducible research. It was attended by approximately 40 participants.
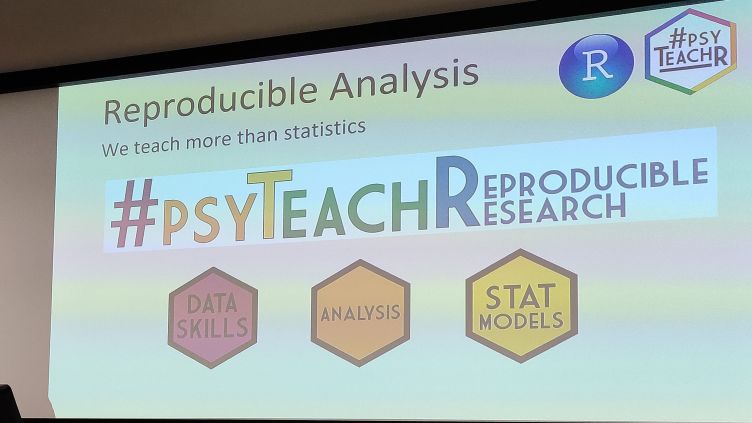
In Carlos’s talk (https://zenodo.org/record/8158825), he spoke about Delft’s commitment to doing science responsibly in a way that maximises the positive benefits to society. This symposium provided an excellent opportunity for us to illustrate with examples how we motivate, and provide practice opportunities to researchers to engage them in learning about the benefits of reproducibility.
The Symposium stimulated interdisciplinary exchange of best practice in doing and teaching open research. The participants discussed different approaches on embedding open science principles in taught programmes in such disciplines, like computer science (Neil Shephard, University of Sheffield), engineering (Alice Pyne, Univ. of Sheffield and Carlos Utrilla Guerrero, TU Delft Library), social sciences (Jenniffer Buckley, Univ. of Manchester and Julia Kasmire, UK Data Service, Jim Uttley, Univ. of Sheffield), geo-data-science (Jon Reades and Andy MacLachlan, UCL), psychology (Marina Bazhydai, Lancaster Univ. and Lisa DeBruine, Univ. of Glasgow). Project TIER’s Directors also gave the first keynote speech arguing in favour of saturating quantitative methods instruction with reproducibility. In the second keynote of the day, Helena Paterson from School of Psychology & Neuroscience at the University of Glasgow reflected on the School journey of redesigning the psychology undergraduate and postgraduate curriculum to focus on teaching reproducible methods and analysis.
On day two, (21 June) Norm and Richard delivered a UKRN accredited workshop (Repository: https://osf.io/3jwyz/) on integrating principles of transparency and reproducibility into quantitative methods courses and research training. During the final day (22 June) Project TIER’s Directors were available for individual and small-group meetings with instructors interested in introducing reproducible methods into their classes.
The conference and workshop was a great opportunity to get to know practices and use cases of embedding open science and reproducible research in teaching activities in different fields. A useful piece of advice from several attendees about effective methods for teaching reproducibility is that today’s students (MSc and BSc) are potentially tomorrow’s researchers, and so integrating reproducibility into the undergraduate curriculum will be crucial in promoting and implementing reproducible practices in the long term. This is in line with the work of the TU Delft Library’s Data Literacy Project, an initiative of the Open Science Programme that launched in March 2023. The Data Literacy Project is investigating how to integrate skills on data literacy and open science practices into the BsC and MsC programmes at TU Delft. Please contact project leader Paige Folsom for more information about the project: P.M.Folsom@tudelft.nl.
Resources:
Event Program: https://www.sheffield.ac.uk/smi/events/teaching-reproducible-research-and-open-science-conference
Presentations: https://drive.google.com/drive/u/0/folders/1uWLbbFscxVsxtdtpcbXz2KWeA417R0so
The programme Booklet: https://issuu.com/smi_events/docs/teaching_reproducibility
RDM 101 openly available as a self-learning resource: https://tu-delft-library.github.io/rdm101-book
The Turing Way Book Dash at TU Delft
Authors: Tanya Yankelevich, Esther Plomp, Julien Colomb

The Turing Way is a ‘lightly opinionated’ online guide to reproducible, inclusive and ethical data science. The book is collaboratively written using GitHub and Jupyter book, an effort led by Kirstie Whitaker and Malvika Sharan.
As part of the Turing Way, we co-organised an event at the TU Delft library! This event was part of the Book Dash that is organised twice a year. During each Book Dash people come together to work on the Turing Way and add their contributions within a short period (hence why it is called a ‘dash’). A large part of the event takes place online, and this year there were in-person hubs in London at the Alan Turing Institute and at TU Delft.

At the TU Delft hub we had several people joining from the Dutch eScienceCenter as well as more internationally based that were interested in Open Hardware to work together to include a more extensive guide on Open Hardware in The Turing Way!
What did we work on?
- Tanya worked on including artists and civil society/community-based organisations as alternative ways for Open Communication of research process and research results. The subsections serve to start the conversation and would benefit from feedback of others with experience with both, artists and civil society/communities, and cases to demonstrate different perspectives.
- Azin got familiar with the Turing Way and had discussions with Barbara on ‘Code linting’.
- Lena worked on Research Data Repositories and Data Feminism (together with Maya, Gigi, Esther), and engaging the general public with art.
- Esther helped Luisa adding a contribution on how to link GitLab and Zenodo, and supported Tanya with her issue and Pull Request, and worked together with Lena on the Research Data Repositories draft. She also reviewed some Pull Requests (#2356), and was able to merge a Pull Request on removing duplication of information in the Open Data and Sensitive Data parts of the book (thanks to Johanna – another online attendee!).
- Carlos worked together with Anne and Alejandro (who joined online) on The Environmental Impact of Digital Research.
- The Open Hardware team (Angela, Santosh, Julien, Sacha, Moritz, Julieta, Nico) worked together on a new Open Source Hardware chapter, including two illustrations.
- Barbara and Pablo worked on an old Pull Request on error message management. During the Dash Barbara commented that “This more than anything makes me believe in Git”, as the pull request was already three years old!
- Julien also worked on data versioning via an old PR, and closed issue 310.

The Delft Hubbers demonstrated a fiery passion about Climate Change in our break discussions (yes, we did try to use the breaks to focus on things other than Book Dash work) and took extra care to follow the Code of Conduct. We promised to work with the university, such that all future events organised at the TU Delft will avoid the use of disposable cups and Nescafé machines… We had a great time getting to know each other in an informal atmosphere and testing the bounds of our biases through jokes. The hub attracted a bunch of Open Science enthusiasts from both the Netherlands and Germany, which inevitably led to a lot of collaboration ideas for the future and future-focused discussions.
The thematic focus (on Open Hardware) was a real success, allowing for specific networking and concrete collaborative work. Informal conversations did naturally sprout the idea to have another thematic focus for a future Book Dash in the Netherlands. A proposed theme is Citizen Science and a guide on “how to Citizen Science”. Thoughts?
Organisation of Research Services department, TU Delft Library
The Research Services department of the Library has recently expanded to four teams, and is appointing some new heads. I have added short descriptions of core tasks for each team
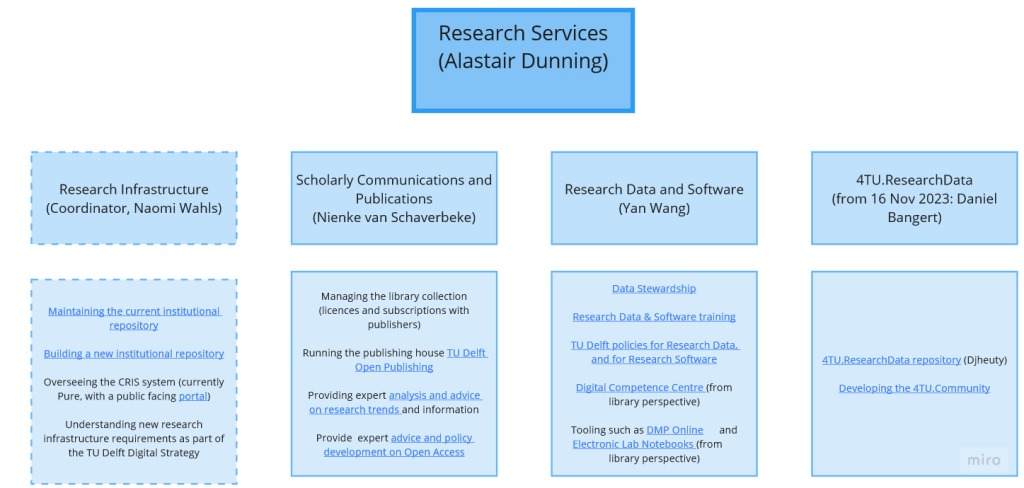
Yan Wang has been appointed Head of Research Data and Software. This team has responsibility for
- Data Stewardship
- Research Data and Software training
- TU Delft policies for Research Data, and for Research Software
- Digital Competence Centre (from library perspective)
- Tooling such as DMP Online and Electronic Lab Notebooks (from library perspective)
Madeleine de Smaele is the Interim Director of 4TU.ResearchData. (We are currently in the recruitment process for a full-time Director) This team has responsibility for the
Naomi Wahls will start on 1 July 2023 Coordinator of Research Infrastructure. The responsibilities of this team include
- Maintaining the current institutional repository
- Building a new institutional repository
- Overseeing the CRIS system (currently Pure, with a public facing portal)
- Understanding new research infrastructure requirements as part of the TU Delft Digital Strategy (eg for Artificial Intelligence, Convergence context)
We are currently recruiting for a Head of Scholarly Communications and Publication. The responsibilities of this team include
- Managing the library collection (licences and subscriptions with publishers)
- Running the publishing house TU Delft Open Publishing
- Providing expert analysis and advice on research trends and information
- Provide expert advice and policy development on Open Access
Update on vacancies: 4TU.ResearchData Director and Coordinator Research Infrastructure
For the position of Coordinator of Research Infrastructure we have received 42 applications. The first round interview process will start in May and end in early June. We have started being in touch with some applicants, and will continue to do this for the next week or so.
For the position of 4TU.ResearchData Director, we have received 16 applications. The first round interviews will take place in 1 and 2 June. We will be in touch with applicants in the week of 15 May.